Policy Lab 1
25th May 2022 – Athens, Greece
The first popAI Stakeholder Policy Lab was held with great success on Wednesday 25 May 2022, coordinated by the National Center for Scientific Research (NCSR) Demokritos, with the decisive support of the Hellenic Police as one of the LEAs (Law Enforcement Authority) of the project.
This event was the first of a series of Policy Labs (6 in total) that will be held in European countries in the coming months aiming to create written recommendations on the ethical regulation and proper use of Artificial Intelligence (AI) for civil protection and citizen security.
During the first Policy Lab, which was held in Greek, 30 entities participated and were involved in discussions from different perspectives and fields of action regarding the development, use and regulation of AI for civil protection and security. They exchanged views and proposed solutions, laying the foundations and the criteria of successful action.
In the workshop participated representatives from the Special Secretariat of Foresight and Research for the Future, the European Union Agency for Asylum, representatives from the Hellenic Police and local government, integrated IT and communications solutions companies, along with legal advisors specialised in Personal Data Protection and AI.
The participants discussed in break-out working groups the possibilities of data exploitation systems for improving policing and dealing with crime as well as traffic accident prevention with the use of video footage presented by the Greek Police in collaboration with IT and communications companies.
By examining the possibilities offered by AI along with the legal, ethical, and social extension of its use – in the context of civil protection – specific recommendations were developed on an operational, legal and technical level. These proposals will be included in the best practices map – produced by the project and will form the basis for the creation of an ecosystem for a sustainable and inclusive European hub on the proper and ethical use of AI by law enforcement agencies (LEAs).
Scenario 1:
Predictive, research, and detection systems using crime data to improve policing and combat crime
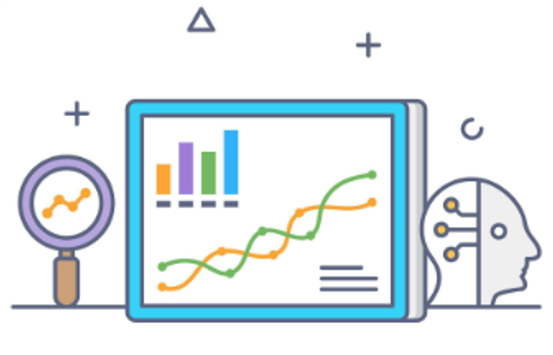
Descriptive, predictive, and prescriptive analytics are different types of analytics models. Within the context of the Policy Lab, it was argued that predictive capabilities based on machine learning (ML) and artificial intelligence (AI) would improve the existing system of crime recording that provides statistics based on collected data including type of crime, location, gender, and age of offender etc.
Databases could be enriched with additional types of data and further parameters creating a new data ecosystem which is not easily processed manually, thus minimising potential human biases. Such an advanced AI system can also advise/support decision-making on different levels of policing.
Key outcomes:
Legal harmonisation of AI usage at the national and European levels – a legal framework to ensure data protection and enable judges’ intervention regarding permission for data usage.
Need for qualified staff/users and model/technology designers to be provided with continuous training processes – relevant certifications to be described in the legal framework.
Interoperability for collaboration between different databases for the best and most effective implementation of the system – for example, recording of unaccompanied minors, foster care cases and adoptions.
Scenario 2:
Systems for predicting dangerous driving using video footage from traffic management cameras or other real-time footage to prevent traffic accidents
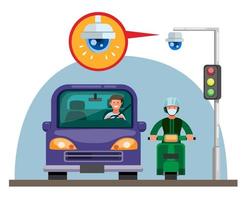
The initial discussion regarded how an AI approach would benefit the prevention of traffic accidents and the ethical dimensions of a potentially emerging mass surveillance system. Profiling and targeting a driver before an accident or highway code offence has been committed is controversial as it is the creation of such a camera network that includes body-worn cameras (as emerged from the discussion).
Key outcomes:
Creation of data intermediaries, i.e. bodies that provide – free of charge – their services to citizens to manage third parties’ data based on their preferences. In this way, when the police use data originally collected for other purposes, citizens will be notified regarding the process, the purpose, storage etc. This process describes the right to informed consent in an automatic way.
Legal frameworks relevant to data protection in the context of the case study: GDPR, AI Act, Data governance act.
Interoperability between crime predictive systems and traffic division for more effective profiling and valid predictions.
The Greek Policy Lab allowed to draw some conclusions and recommendations at organisational, regulatory and technical levels, while offering food for thoughts for the remaining Policy Labs.
The discussions pointed out other relevant considerations: definitions are crucial in a context where different sectors interact and collaborate, as the same expression could have specific meanings depending on the field of expertise.
Participants concluded that in both case studies a greater focus should be the social benefit.
In the first case, for example, the system could be used to identify the potential correlation between financial difficulties and domestic violence. Therefore, it could assist organisations in predicting but also acting preemptively and guiding policy-making through evidence-based approach. In the second context, the system could be interoperable with other governmental bodies. The system could produce statistical reports and provide specific classifications (e.g., traffic accidents signalling a lack of infrastructure) that can indicate potential causes of accidents. Consequently, such a system should be considered in the context of an interdisciplinary urban planning debate on the foresight of system evolution and development – evaluation and use of algorithms for future systems.
Agenda of the meeting
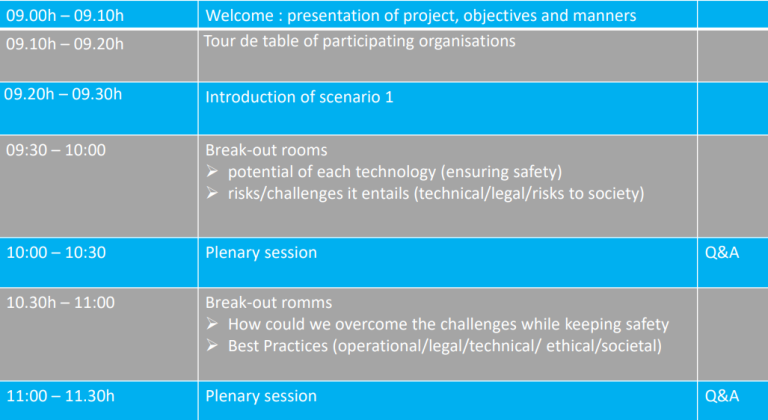
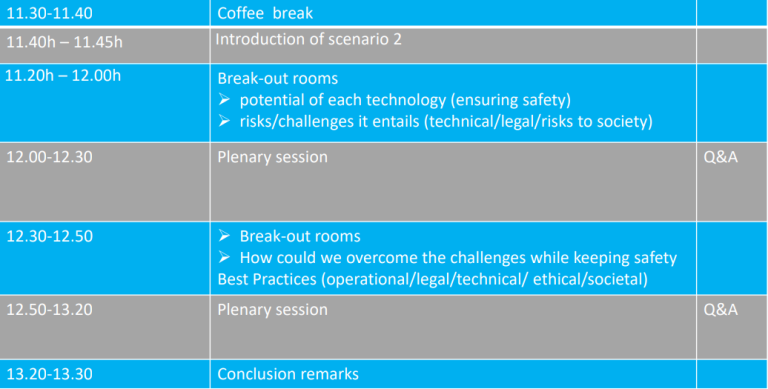